A new paper titled “Augmentation of Fingerprints for Indoor BLE Localization Using Conditional GANs” by Suhardi Azliy Junoh and Jae-Young Pyun, explores the development of a data-augmentation method for enhancing the accuracy of indoor localisation systems that use Bluetooth Low Energy (BLE) fingerprinting.
Bluetooth fingerprinting is a technique used to identify and track devices based on the unique characteristics of the Bluetooth signal, such as hardware addresses and signal strength, at specific locations.
The primary challenge addressed is the labour-intensive and expensive nature of traditional site surveys required for collecting Bluetooth fingerprints. The authors propose a novel approach that employs a Conditional Generative Adversarial Network with Long Short-Term Memory (CGAN-LSTM) to generate high-quality synthetic fingerprint data. This method aims to complement existing fingerprint databases, thereby reducing the need for extensive manual site surveys.
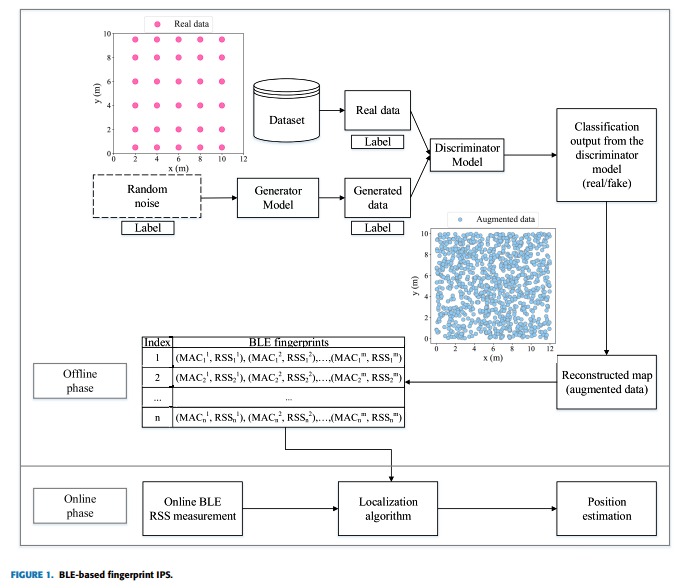
The research found that augmenting the fingerprint database using the CGAN-LSTM model significantly improved localisation accuracy. In experimental evaluations, the proposed data augmentation framework increased the average localization accuracy by 15.74% compared to fingerprinting methods without data augmentation. Moreover, when compared to linear interpolation, inverse distance weighting, and Gaussian process regression, the proposed CGAN-LSTM approach demonstrated an average accuracy improvement ranging from 1.84% to 14.04%, achieving average accuracies of 1.065 and 1.956 meters in two different indoor environments. These results underline the effectiveness of the CGAN-LSTM model in capturing the complex spatial and temporal patterns of BLE signals, making it a promising solution for indoor localisation challenges.
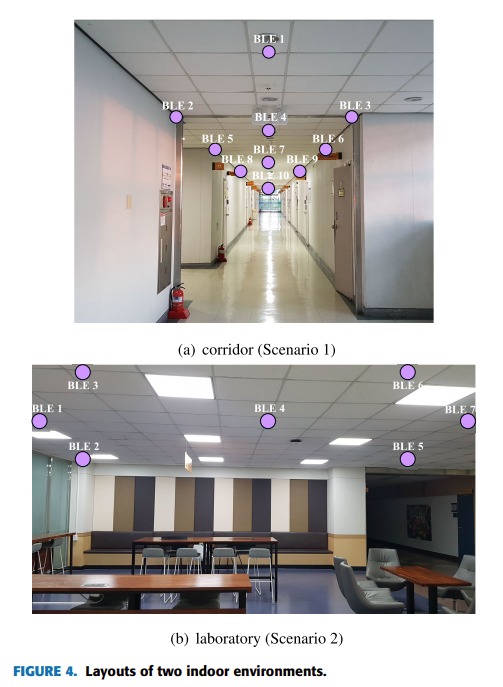
The study contributes significantly to the field by demonstrating how synthetic data can enhance the performance of fingerprint-based localisation systems in a cost-effective and efficient manner. The authors suggest that this approach could alleviate the burdensome demands of manual site surveys, offering a viable solution for improving the accuracy of BLE-based indoor localisation while minimizing resource expenditure.