Sensor beacons provide a quick and easy way to obtain data for AI machine learning. They provide a way of measuring physical processes to provide for detection and prediction.
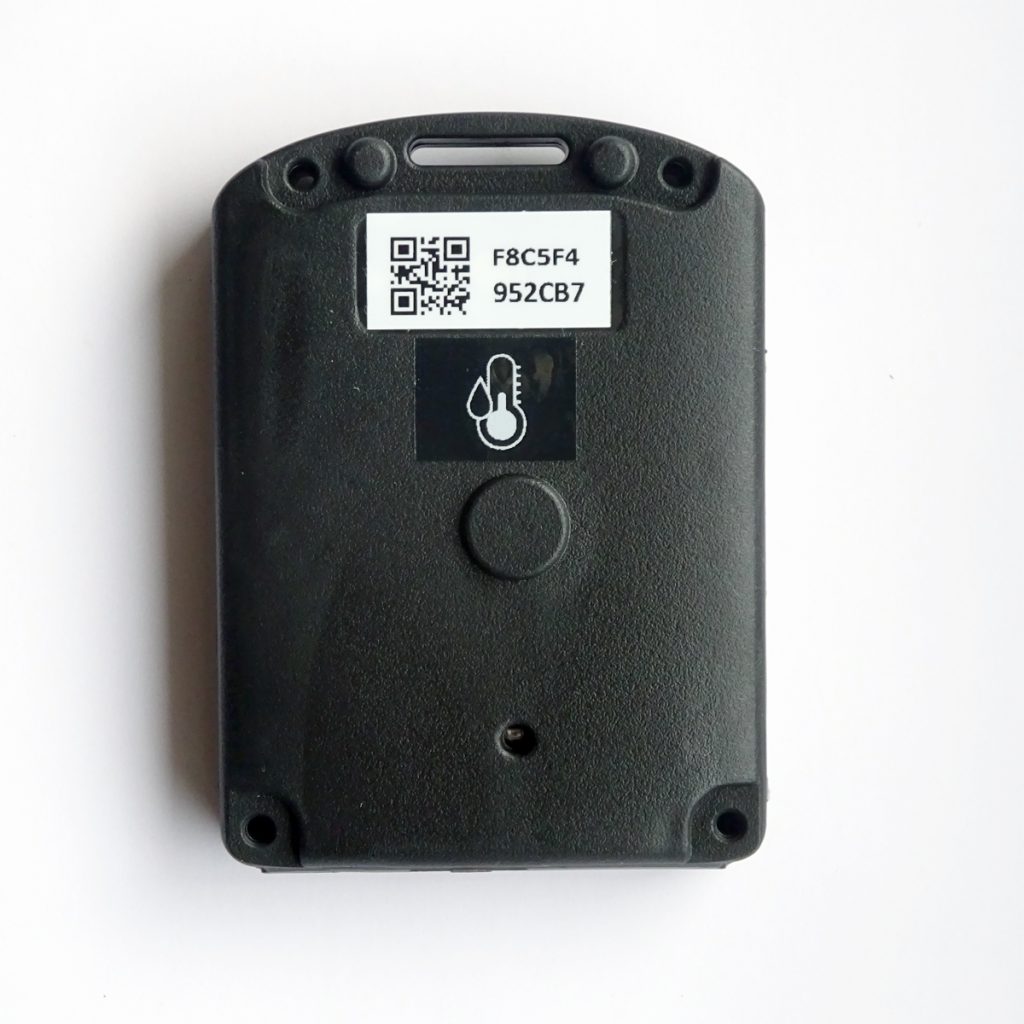
Beacons detect movement (accelerometer), movement (started/stopped moving), button press, temperature, humidity, air pressure, light level, open/closed (magnetic hall effect), proximity (PIR), proximity (cm range), fall detection, smoke and natural gas. The open/closed (magnetic hall effect) is particularly useful as it can be used on a multitude of physical things for scenarios that require digitising counts, presence and physical status.
The data is sent via Bluetooth rather than via cables which means there’s no soldering or physical construction. The Bluetooth data can be read by smartphones, gateways or any devices that have Bluetooth LE. From there it can be stored in files for reading into machine learning.
Such data is often complex and it’s difficult for a human to devise a conventional programming algorithm to extract insights. This is where AI machine learning excels. In simple terms, it reads in recorded data to find patterns in the data. The result of this learning is a model. The model is then used during inference to classify or predict situations based on new incoming data.
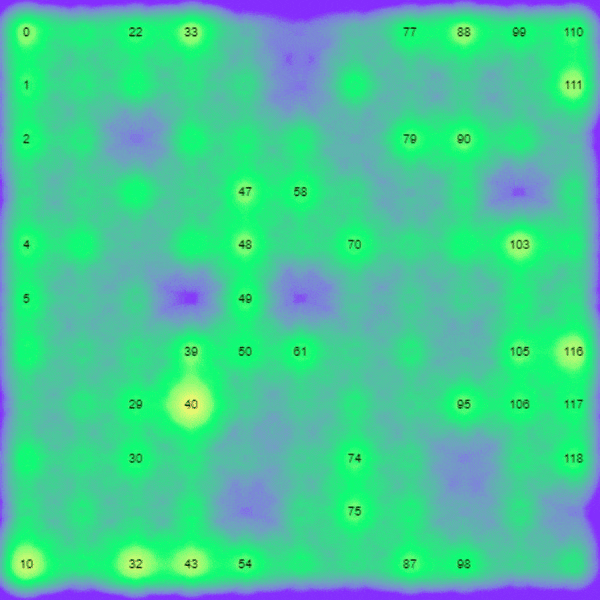
The above shows some output from accelerometer data fed into one of our models. The numbers are distinct features found over the time series as opposed to a single x,y,z sample. For example ’54’ might be a peak and ’61’ a trough. More complex features are also detectable such as ‘120’ being the movement of the acceleration sensor in a circle. This is the basis for machine learning classification and detection.
It’s also possible to perform prediction. Performing additional machine learning (yes, machine learning on machine learning!) on the features to produce a new model tells us what usually happens after what. When we feed in new data to this model we can predict what is about to happen.
The problem with sensor data is there can be a lot of it. It’s inefficient and slow to detect events when this processing at the server. We create so called Edge solutions that do this processing closer to the place of detection.